Do ice cream sales lead to higher crime rates?
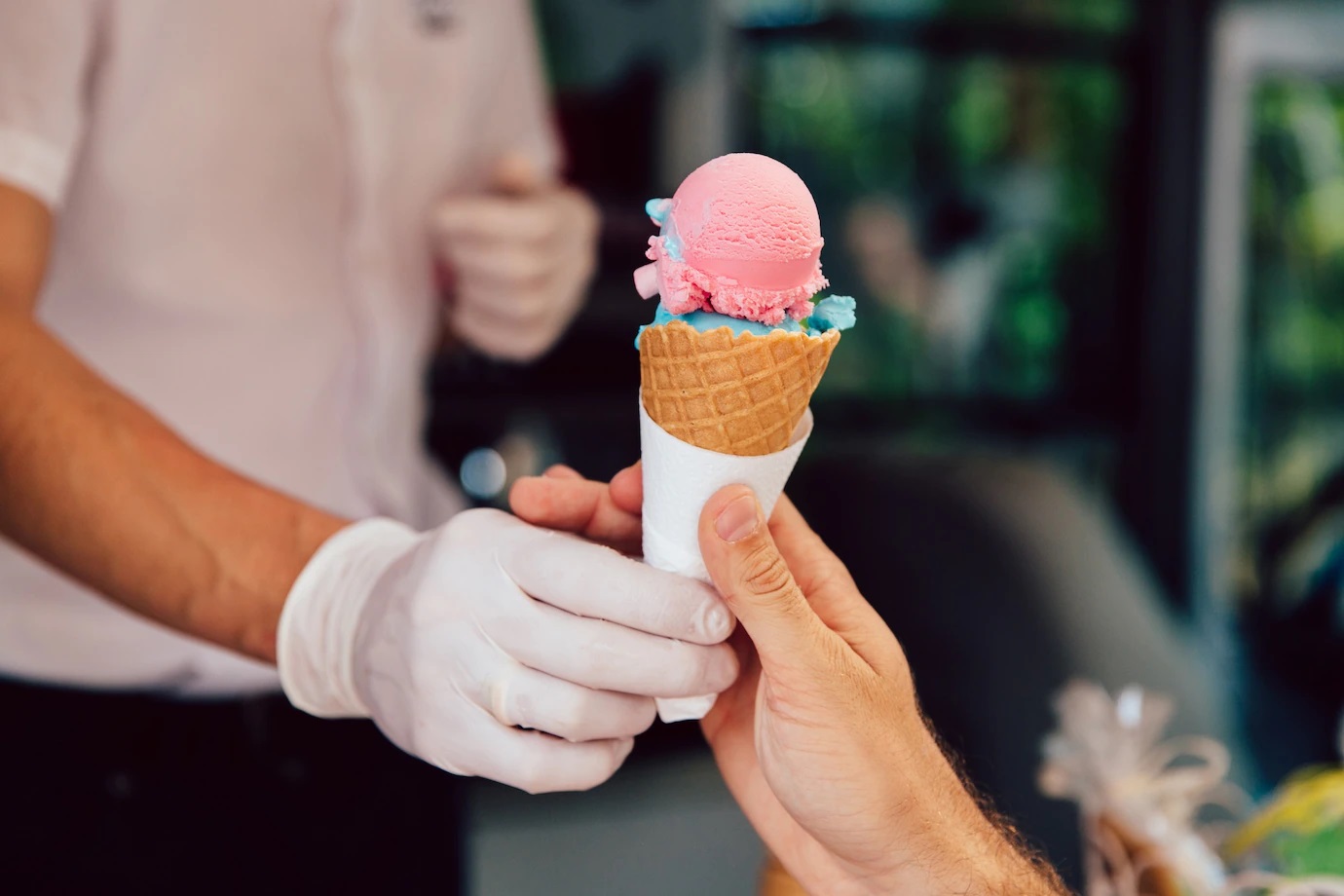
By Wei Guo
Can you trust your empirical intuitions?
Today, many companies use fancy spreadsheets to present data. However, distilling valuable information from spreadsheets is becoming increasingly difficult.
In many cases, available data narrowly focuses on a limited range of factors and thus fails to tell a complete story. Additional time is required to fully understand what the data means. Consequently, despite having data at hand, many companies choose to revert to their old ways, relying upon intuition. The reason is simple: Judgements based on intuition can be made in a heartbeat. Companies want to reach a decision as quickly as possible. In short, time is everything.
For example, before making a decision, companies usually embark on market research, which involves data collection, data interpretation, and the compilation of reports. This entire process requires considerable time and effort. As a result, for the sake of efficiency, many companies instead opt for quick decisions based on experience and intuition.
However, decisions derived from prior experience or intuition can be accompanied by shortcomings. For starters, intuitive judgements are prone to uncertainties. Companies can never be sure whether their prior experience will still be applicable under future circumstances. More importantly, human beings are inescapably prone to confirmation biases — the tendency to favour information that confirms or supports our prior beliefs and ignore contrary information. In other words, we often overestimate what we know and believe, underestimate what we don’t believe, and mistake our own opinions for “facts”.
Confirmation Biases
Let’s do a self-check using the following two questions:
Q1: Does the English language contain more words beginning with the letter k or more words with k as the third letter?
A) More words beginning with the letter k
B) More words with k as the third letter
Answer: B
Q2: Six hundred people are infected with a deadly disease. Which of the following treatments would you choose?
Treatment A) Has a 100% chance of saving 200 lives
Treatment B) Has a 33% chance of saving 600 lives, but with a 66% chance that everyone dies
Answer: The two treatments save the same number of lives (Treatment A = 200 * 100% = 200 and Treatment B = 33% * 600 + 0% * 0 = 200)
Five common types of cognitive biases
What were your answers to the above questions? Did you get them right? When making decisions, we are subject to different types of biases that we are often not aware of. Here is an overview of five common types of cognitive biases:
- Availability heuristic
The availability heuristic is about making judgments based on information that is more easily recalled (i.e., more available in our memories). If certain memories or examples are readily available, we presume them to be commonplace. For example, let’s suppose you have experienced flight delays in the past and constantly hear complaints about delayed flights. You may thus conclude that a high percentage of flights are delayed, which might not be the actual case. When estimating the likelihood of an event, we need to examine overall statistics from a representative sample.
- Availability cascade
The availability cascade explains how information is perceived to be true and correct due to its increasing frequency of repetition in the public domain. Take, for example, coverage of violence and crimes in the media. Horrid crimes easily spark media attention and public discourse, which may lead to the perception that such crimes are widespread and frequent.
- Anchoring effect
The anchoring effect describes the common human tendency to rely too heavily on the first piece of information offered. Retailers, for example, take advantage of this cognitive bias in their pricing strategies. When selling a product at $8.70, instead of plainly stating $8.70, the retailer may include an initial struck-out price of $9.90 and display a “discounted price” of $8.70. The “initial price” of $9.90 serves as an anchor that influences consumers’ perceptions of the value of the product. When they think they are getting a higher-value product at a discounted price, consumers are more likely to make a purchase.
- Illusion of knowledge
When we know about something, we tend to expect others to know it too, and we find it hard to imagine someone else who doesn’t know about it. An example of this bias was demonstrated in an experiment in which psychologists separated college students with a passion for NBA into two groups. Group A was given statistical information (such as the number of games a certain team won during the season and the scores of previous games), but not the name of the team. Group B was given the same statistics plus specific knowledge about the team (e.g. its name, history, and team members).
Both groups were then asked to predict the team’s performance in the upcoming game. So, which group do you think would produce a more accurate prediction? The results suggest that Group A (with less information) made more accurate predictions. Group A’s predictions were based purely on statistical information and were more objective. Group B, knowing which team they were making predictions about, was inevitably influenced by subjective judgements or even emotional factors. The illusion of knowledge led to poorer predictions.
- Endowment effect
The endowment effect describes a circumstance in which people place greater value on an object they own than on one they don’t. The endowment effect leads to asymmetries in valuations and exchange behaviour. When selling something they own (e.g., one’s house), individuals tend to charge a price that is higher than the market value.
Do ice cream sales lead to higher crime rates?
Since human beings are continually under the influence of cognitive biases, rational decisions should not be made based on intuitions. Data-driven decision-making (or DDDM) can help companies to rid themselves of cognitive biases. DDDM refers to decision-making processes that place factual data before intuitive judgements or subjective observations.
According to a report titled Gut & Gigabytes: Capitalising on the Art & Science of Decision Making by the Economist Intelligence Unit, 43% of C-suite executives surveyed believe that highly data-driven companies are more likely to report improvements in big decision making in the previous two years. Specifically, highly data-driven companies are three times as likely to report “significant” improvements in big decision making when compared with peers who are not highly data-driven, the survey suggests.
Once we recognise the effectiveness of data-driven decisions, what can we do in our data analysis to support DDDM?
First of all, we need to understand that the availability of data does not directly translate to effective decisions. When analysing the data, we need to bear in mind the following questions:
- What happened? What is happening (phenomenon)?
- Why did it happen (cause)?
- Will it happen again?
- What will trigger this event to happen again (necessary and sufficient conditions)?
- If the conditions are altered, will the event still happen?
Now, consider the following question: Do ice cream sales lead to higher crime rates? Statistics indicate a correlation between homicides and ice cream sales. Nevertheless, few of us would assume that if we took away all of the ice cream, crime rates would drop to zero. When examining a statistical correlation, we should explore the reason behind the phenomenon. Why did it happen? What might be the sociological and psychological explanation?
The next question we would want to ask is this: What would stop the event from happening? Will the suspension of ice cream sales stop crimes? Apparently not. Correlation is not causation. That said, it stands to reason that ice cream sells better in warm weather, and there is in fact plenty of evidence to suggest that crime rates rise when temperatures rise. In the summer, the sun sets late, and people stay out late. In addition, many prefer to leave their windows open for better ventilation (which might be taken advantage of by criminals).
The ice cream example demonstrates the importance of digging deeper into data to understand what has caused observed trends or correlations. Below are three types of analytical methods that can be used in data processing:
- Descriptive analytics: A statistical visualisation of what has happened (e.g., a chart that illustrates past sales numbers).
- Predictive analytics: A method that creates forecasts by feeding historical data into a model to identify key influential factors, trends, or patterns (e.g., regression analysis and supervised and unsupervised machine learning).
- Prescriptive analytics: An optimisation tool that simulates the potential outcomes of different decision options.
Should Google get rid of its middle managers?
As an example, let’s look at how Google has applied the above theories in real life.
Google is widely known as “a company built by engineers for engineers.” Its two co-founders, Larry Page and Sergey Brin, both started out as engineers. Given its highly technocratic culture, employees of Google had long questioned the value of the company’s managers.
In 2002, Google experimented with a completely flat organisational structure, eliminating managers in an effort to improve internal operations. That experiment lasted only a few months, because the company was in chaos after all of the managers were removed.
Why did this happen? Because Google’s engineers were problem-solvers who preferred “real work” over project management. All of them focused on their separate tasks and goals. Without the coordination effected through their managers, engineers worked in silos on specific projects. The company as a whole was not moving anywhere.
The experiment revealed to Google the importance of managers. Google then set out to design another test to determine when and why managers are important.
The follow-up initiative took the form of double-blind qualitative interviews. Managers were asked questions such as “How do you manage your team?” and “What are the things you usually do at work?” Yet, during each interview, neither the interviewer nor the interviewee knew whether the manager was a high-scoring or low-scoring manager. After information was collected and processed, eight key attributes demonstrated by the company’s most effective managers were identified:
What Google’s Best Managers Do
Google’s story is a great example of how a globally renowned company makes data-driven decisions. We can definitely take a page from Google’s book and explore areas of improvement in our own organisations.
A five-step approach to data analysis:
- Convert a business problem into an analytical question
- Collect data
- Make intuitive observations of the data
- Analyse data and identify causes
- Develop data-based solutions for the initial business problem
Guo Wei is an Associate Professor of Strategy and Entrepreneurship at CEIBS. For more on her teaching and research interests, please visit her faculty profile here.